Xinyu Yuan, a master's student from the School of Computer Science and Information Engineering at Hefei University of Technology, under the guidance of Associate Professor Yan Qiao, has had his research paper titled “Diffusion-TS: Interpretable Diffusion for General Time Series Generation” accepted and published at The Twelfth International Conference on Learning Representations (ICLR 2024). Professor Yan Qiao is the corresponding author of the paper. ICLR, founded in 2013 by deep learning pioneers such as Yoshua Bengio and Yann LeCun, is one of the top three machine learning conferences alongside NeurIPS and ICML. Papers published in ICLR have a profound impact on both academia and industry, ranking second on Google Scholar's h5-index for engineering and computer science, and AI-related journals/conferences with a score of 304.
Paper Title: Diffusion-TS: Interpretable Diffusion for General Time Series Generation
Authors: Xinyu Yuan and Yan Qiao
Paper Link: openreview.net/pdf?id=4h1apFjO99
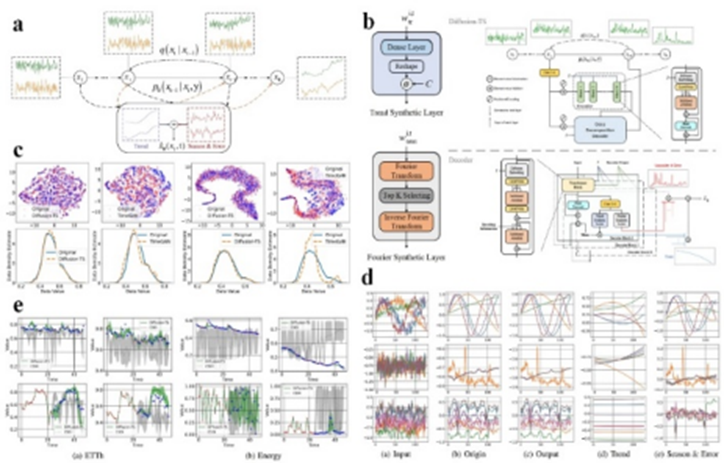
Figure 1
a. Important Information in Time Series: The key information in time series (such as periodicity and trends) is gradually lost during the noise-adding process in the diffusion model, making it necessary to incorporate expert knowledge to guide the generation process during the reverse restoration.b. Diffusion-TS Framework: The underlying model of Diffusion-TS can essentially be viewed as a deep decomposition network based on a Transformer encoder-decoder architecture. After the encoder extracts useful information from the noisy sequence, the decoder reconstructs the trend and periodicity of the time series through special constraints (polynomial and inverse Fourier transform).c. Advantage in Distribution Learning and Alignment: Diffusion-TS outperforms mainstream GAN-based time series generation models in distribution learning and alignment.d. Model Interpretability: The outputs of the model's individual modules, namely the trend synthesis module and the periodicity & residual synthesis module, are highly interpretable.e. Performance in Conditional Generation Tasks: The unconditional generation method proposed in this paper also outperforms dedicated conditional diffusion models in tasks such as imputation and prediction.
Diffusion models are becoming a mainstream paradigm for generative models. In this paper, the authors propose a novel diffusion-based framework, Diffusion-TS, which uses an encoder-decoder Transformer with decoupled time representations to generate high-quality multivariate time series samples. This framework guides Diffusion-TS to capture the semantic information of time series using decomposition techniques while mining detailed sequence information from noisy model inputs through the Transformer. Unlike existing diffusion-based methods, Diffusion-TS is expected to generate time series that are both interpretable and realistic. The study also shows that Diffusion-TS can easily scale to conditional generation tasks, such as prediction and imputation, without any modification to the model. This motivates further exploration of Diffusion-TS's performance in irregular settings. Finally, qualitative and quantitative experiments demonstrate that Diffusion-TS achieves state-of-the-art performance on various practical time series analysis tasks.